Stefan Wager on Why Your Machine Learning Solves The Wrong Problem
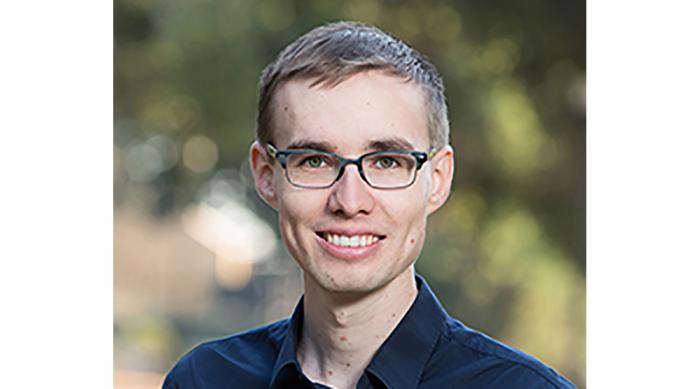
Stanford University
Stefan Wager is an associate professor of operations, information and technology at Stanford Graduate School of Business, and an associate professor of statistics (by courtesy). He received his PhD in statistics from Stanford University in 2016, and also holds a BS (2011) degree in mathematics from Stanford.
He was a postdoctoral researcher at Columbia University during the academic year 2016–2017, and has worked with or consulted for several Silicon Valley companies, including Dropbox, Facebook, Google, and Uber.
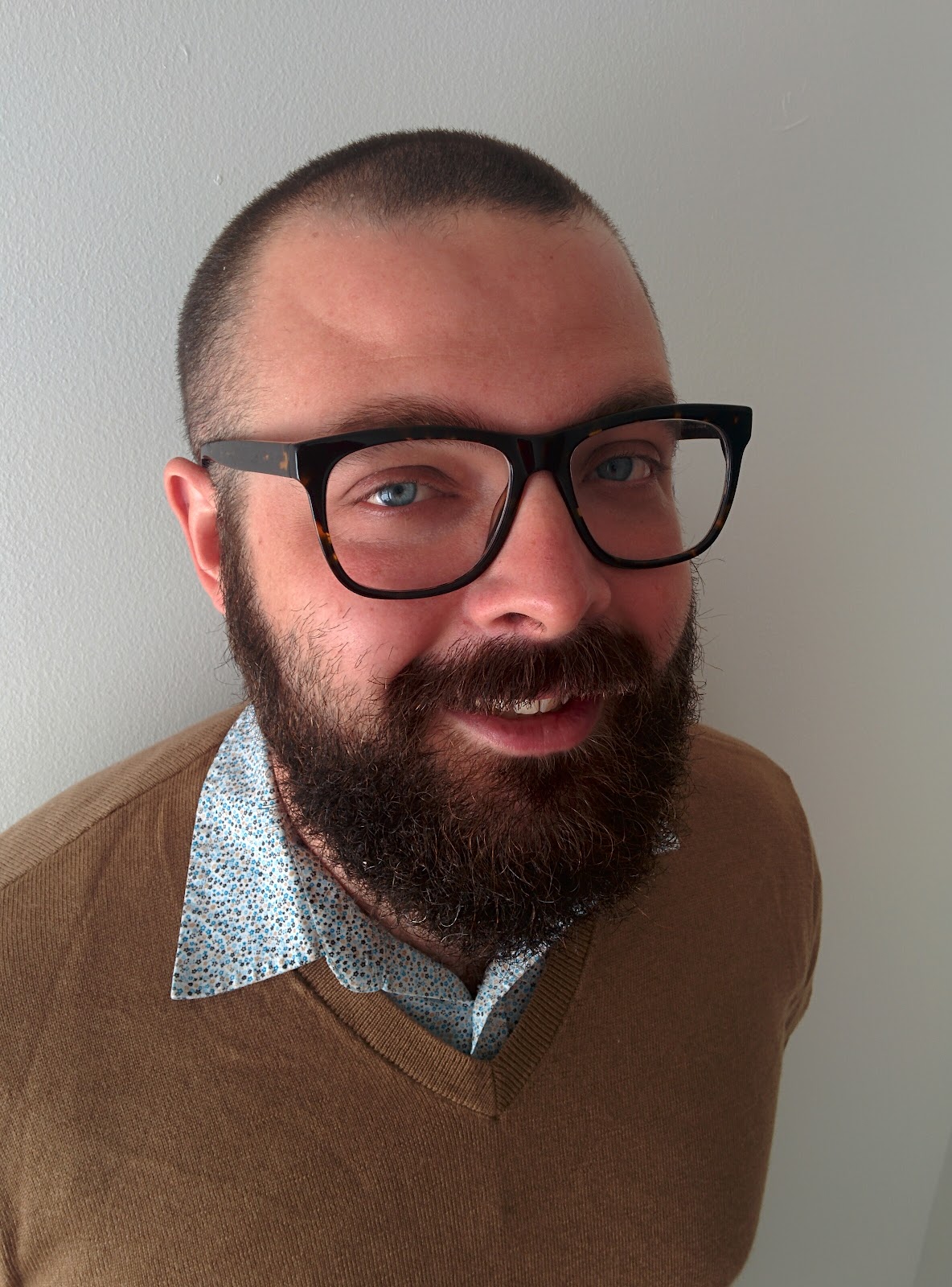
Delphina
Hugo Bowne-Anderson is an independent data and AI consultant with extensive experience in the tech industry. He is the host of the industry podcast Vanishing Gradients, a podcast exploring developments in data science and AI. Previously, Hugo served as Head of Developer Relations at Outerbounds and held roles at Coiled and DataCamp, where his work in data science education reached over 3 million learners. He has taught at Yale University, Cold Spring Harbor Laboratory, and conferences like SciPy and PyCon, and is a passionate advocate for democratizing data skills and open-source tools.
Key Quotes
Key Takeaways
1. Machine Learning Predicts, but Decisions Require Causation
Classical ML excels at predicting the status quo—but most organizations aren’t trying to predict the world, they’re trying to change it. Stefan explains why prediction alone fails at critical “what-if?” questions.
2. Beware of Churn Prediction Pitfalls—Use Experiments to Find Real Impact
Predicting churn probability alone won’t help you choose effective interventions. Stefan shares concrete examples from industry—like loyalty programs—where predictive models have led companies astray, and shows how causal ML corrects these mistakes.
3. Experiments Are Crucial—and Easy to Get Wrong
Causal ML depends heavily on experimental data. Stefan highlights common pitfalls in running experiments, such as accidental biases that invalidate results. Getting experimentation right is fundamental to success.
4. Start with Clarity—Define Actions, Not Just Predictions
The hardest part of causal ML isn’t running models, but clearly defining the decisions you want to make. Stefan emphasizes that actionable questions must drive data collection, modeling choices, and interpretation.
5. Causal ML Requires Rethinking the ML Workflow
To effectively deploy causal ML, you need to move beyond traditional “XY” prediction workflows and integrate explicit causal reasoning into data collection, model development, and business strategy.
5. Tools Matter, but Thinking Matters More
Stefan suggests mastering just a few powerful causal ML tools—like generalized random forests—rather than chasing every new method. But he emphasizes that good causal inference always starts with careful, strategic thinking about your business problem.
You can read the full transcript here.
00:00 The Limitations of Prediction
01:08 Causal Machine Learning: A New Approach
04:20 Introducing Stefan Wager
04:23 The Importance of Causal Inference
07:31 Challenges and Adoption in Industry
15:52 Practical Examples and Case Studies
20:25 Implementing Causal ML in Organizations
25:14 The Value of Experiments in Causal Analysis
25:34 Challenges with Observational Data
26:12 Industry's Approach to Causal Inference
27:11 Historical Examples and Model Evaluation
28:30 Heuristics for Choosing Modeling Techniques
28:56 Tree-Based Methods and GRF Software
33:27 Communicating Causal ML Results
37:00 Explainable ML vs. Causal ML
40:37 Causal Discovery in Different Fields
42:44 Failure Modes in Causal ML
45:03 Industry vs. Academia in Causal ML
49:18 Resources for Learning Causal Inference
50:43 Future of Causal ML in Business
52:08 Final Thoughts and Common Sense in ML
Links From The Show
- Stefan's Stanford Website
- Machine Learning and Economics, Stefan and Susan Athey's lectures for the Stanford Graduate School of Business
- Causal Inference: A Statistical Learning Approach (WIP!)
- Mastering ‘Metrics: The Path from Cause to Effect by Angrist & Pischke
- The Book of Why: The New Science of Cause and Effect by Judea Pearl and Dana Mackenzie
- Causal Inference: The Mixtape by Scott Cunningham
- A Technical Primer On Causality by Adam Kelleher
- What Is Causal Inference? An Introduction for Data Scientists by Hugo Bowne-Anderson and Mike Loukides
- The Episode on YouTube
- Delphina's Newsletter
Transcript
In the spotlight: Our most popular episodes
Listen up: Our latest discussions
Hear the hottest takes on data science and AI.
Get the latest episodes in your inbox
Never miss an episode of High Signal by signing up for the Delphina newsletter.