Ari Kaplan on Why AI Won't Save You But Data Intelligence Will

Databricks
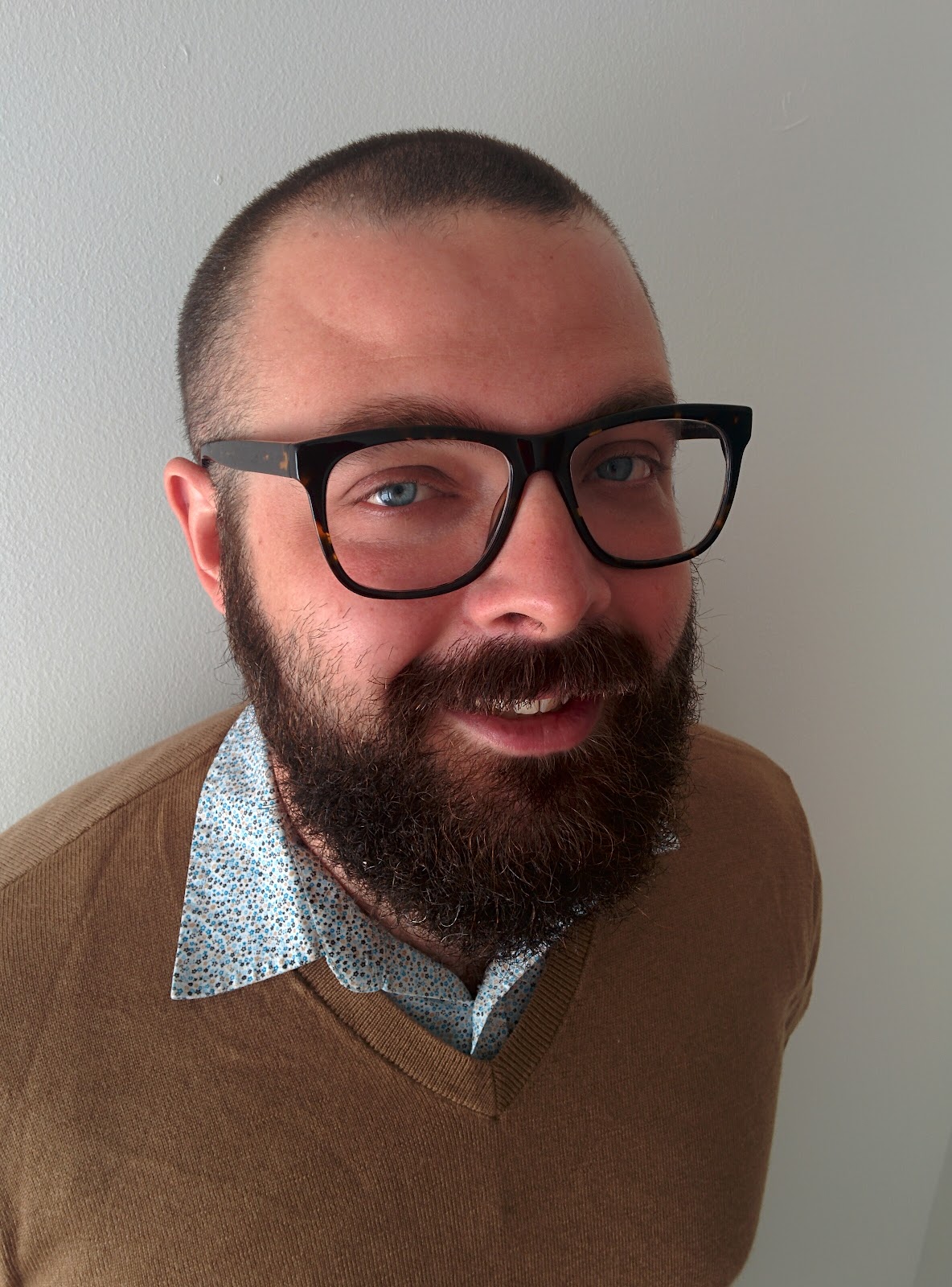
Delphina
Hugo Bowne-Anderson is an independent data and AI consultant with extensive experience in the tech industry. He is the host of the industry podcast Vanishing Gradients, a podcast exploring developments in data science and AI. Previously, Hugo served as Head of Developer Relations at Outerbounds and held roles at Coiled and DataCamp, where his work in data science education reached over 3 million learners. He has taught at Yale University, Cold Spring Harbor Laboratory, and conferences like SciPy and PyCon, and is a passionate advocate for democratizing data skills and open-source tools.
Key Quotes
Key Takeaways
1. Baseball Changed Its Rules Because of Data—Most Businesses Still Ignore It
Defensive shifts in MLB went from 3% to over 50% of plays, forcing the league to ban the strategy entirely. The Cubs didn’t get better players—they just used better data. This kind of shift is possible in every industry, but most companies still aren’t taking full advantage of their own data.
2. AI Models Are a Commodity—Your Data Pipeline Isn’t
Every company has access to the same foundation models, yet many believe AI will give them a unique edge. The real differentiator is how well companies organize and extract insights from their own data. Instead of chasing the latest GPT model, the real investment should be in data intelligence and infrastructure.
3. Most AI Teams Waste Time Chasing Models Instead of Fixing Data Retrieval
Businesses obsess over model upgrades, but Kaplan has seen companies get bigger performance gains from improving OCR, chunking strategies, and retrieval pipelines. AI success isn’t about having the best model—it’s about feeding models structured, high-signal data.
4. 2025 Won’t Be the Year of AI Agents—It’ll Be the Year We Watch Them Fail
Everyone is hyping up agentic AI systems, but most companies will struggle with failure modes, debugging, and business logic. Expect more hard lessons about why agents break than success stories. The real winners will be those who focus on reliability over raw automation.
5. Data Intelligence Will Automate Decision-Making—But You Still Need Humans to Stop Bad Calls
Kaplan worked on sports analytics systems that recommended lineup changes, but coaches still had to decide when to override the data. The same is true for AI in business—automation helps, but blindly trusting models leads to bad decisions. Keeping humans in the loop is essential for catching edge cases and strategic missteps.
You can read the full transcript here.
00:00 The Impact of Data on Baseball Strategy
00:45 Introduction to Ari Kaplan and His Work
01:10 Exploring AI vs. Data Intelligence
01:33 Sponsorship and Podcast Information
01:50 Ari Kaplan's Role at Databricks
02:56 The Evolution of Data and AI at Databricks
03:41 The Importance of Data Intelligence
06:37 Challenges and Metrics in AI and Data Science
15:15 Automation in Data Science and Machine Learning
20:24 The Role of Human Expertise in AI
30:34 The Rise of Data Intelligence
32:48 Human Rights Work and Gen AI
33:55 A Journey Through Sports Analytics
37:15 The Evolution of Baseball Analytics
40:10 Travis Kelsey and the Power of Details
44:43 Advice for Data Science Leaders
49:17 The Future of Data Intelligence
51:18 Tools and Processes for Data Accessibility
54:29 The Role of Human Judgment in Automation
58:57 Conclusion and Final Thoughts
Links From The Show
Transcript
In the spotlight: Our most popular episodes
Listen up: Our latest discussions
Hear the hottest takes on data science and AI.
Get the latest episodes in your inbox
Never miss an episode of High Signal by signing up for the Delphina newsletter.