Ramesh Johari on How to Build an Experimentation Machine and Where Most Go Wrong

Stanford University
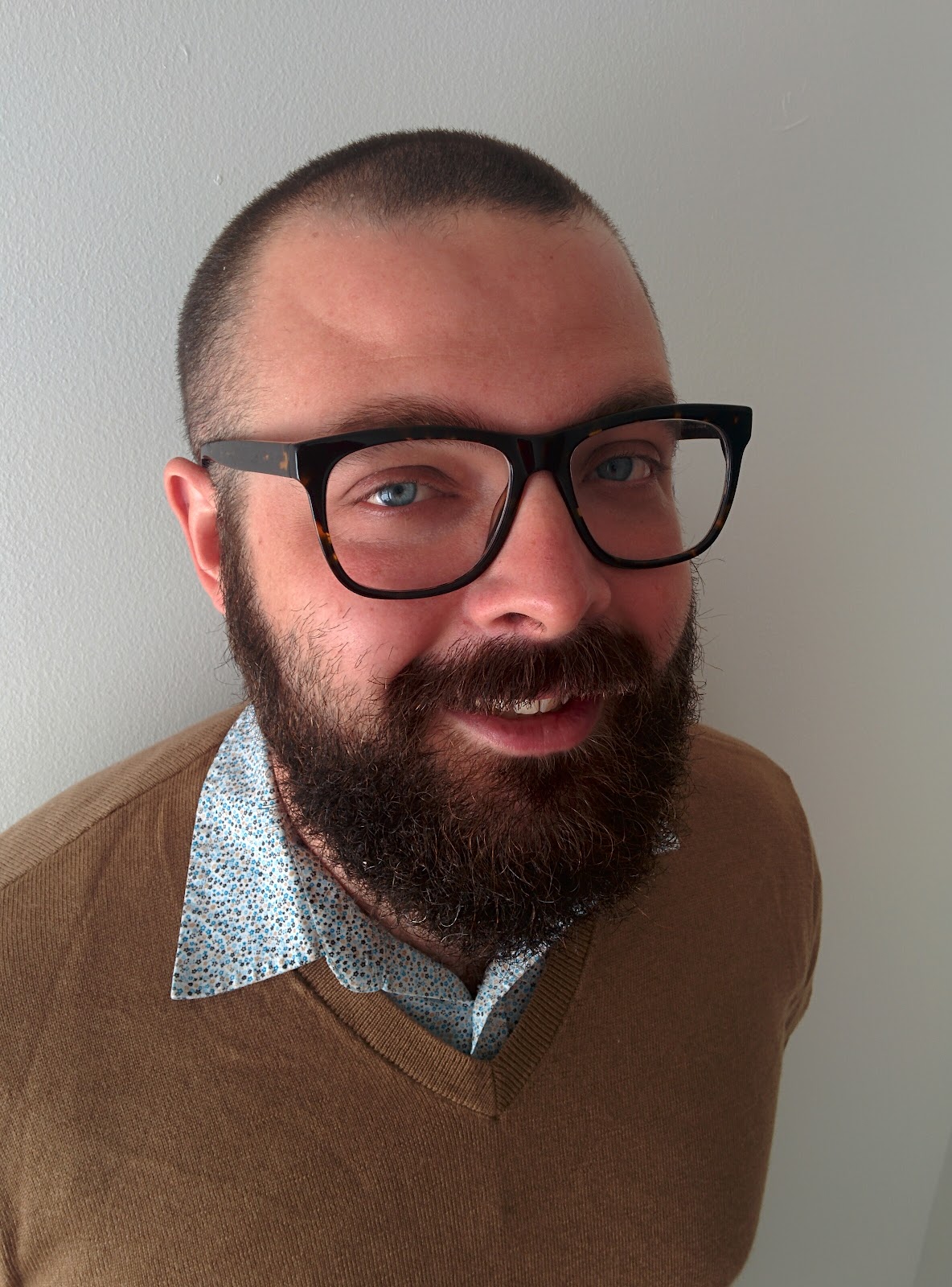
Delphina
Hugo Bowne-Anderson is an independent data and AI consultant with extensive experience in the tech industry. He is the host of the industry podcast Vanishing Gradients, where he explores cutting-edge developments in data science and artificial intelligence.
As a data scientist, educator, evangelist, content marketer, and strategist, Hugo has worked with leading companies in the field. His past roles include Head of Developer Relations at Outerbounds, a company committed to building infrastructure for machine learning applications, and positions at Coiled and DataCamp, where he focused on scaling data science and online education respectively.
Hugo's teaching experience spans from institutions like Yale University and Cold Spring Harbor Laboratory to conferences such as SciPy, PyCon, and ODSC. He has also worked with organizations like Data Carpentry to promote data literacy.
His impact on data science education is significant, having developed over 30 courses on the DataCamp platform that have reached more than 3 million learners worldwide. Hugo also created and hosted the popular weekly data industry podcast DataFramed for two years.
Committed to democratizing data skills and access to data science tools, Hugo advocates for open source software both for individuals and enterprises.
Key Quotes
Key Takeaways
1. The Evolution of Experimentation in Organizations
- Organizations mature from simple A/B testing to becoming self-learning systems.
- Moving beyond "winner/loser" tests involves focusing on why things work or fail.
- Practical Tip: Don't just measure conversions; analyze underlying user behavior to uncover root causes of successes or failures.
2. Risk Aversion and the Need for Speed
- Risk-averse cultures often overanalyze experiments, leading to fewer, slower tests.
- High-velocity organizations prioritize frequent, incremental testing to reduce the stakes of any single experiment.
- Practical Tip: Use phased rollouts and real-time monitoring to minimize the risk of large-scale changes.
- Practical Tip: Create systems where even small teams can run experiments autonomously, democratizing experimentation.
3. The Role of Generative AI in Experimentation
- Generative AI enables rapid hypothesis generation, creating 10x more ideas to test.
- This requires systems to synthesize insights across multiple experiments to avoid data overload.
- Practical Tip: Use AI tools to automatically detect patterns across experiments and generate new hypotheses based on past results.
- Practical Tip: Balance the volume of ideas generated with the organization’s ability to test and implement them quickly.
4. Data Over Methods
- High-quality data is more impactful than complex statistical methods or algorithms.
- Experimentation is most effective when data informs clear, actionable decisions.
- Practical Tip: Avoid overcomplicating analysis; prioritize simple, interpretable methods like A/B testing over advanced techniques unless necessary.
- Practical Tip: Ensure all teams have access to clean, reliable data and encourage sharing insights across the organization.
5. The Vision of a Self-Learning Organization
- A self-learning organization runs experiments continuously, learns from them, and adapts dynamically.
- Experimentation in such organizations isn’t limited to product decisions but informs overall strategy.
- Practical Tip: Build feedback loops where test results directly influence future ideas.
- Practical Tip: Treat every test as a step in a broader strategy, rather than a standalone decision.
You can read the transcript here.
Timestamps:
0:00 Introduction
1:00 Episode Overview
3:00 The Future of Experimentation
6:00 Meet the Team at Delphina
7:30 Ramesh’s Background
9:30 What is Experimentation?
12:00 Evolution of Experimentation in Organizations
16:00 The Risk Aversion Cycle
18:00 Incentives in Experimentation
20:00 Fat Tails in Experimentation
24:00 The Role of Dogfooding
26:00 Experimentation and Prediction
29:00 Encouraging a Culture of Experimentation
32:00 Embedding Data Scientists
34:00 Generative AI and Experimentation
38:00 AI’s Role in Managing Experimentation Data
40:00 The Self-Learning Organization
42:00 Limitations of Experiments
46:00 Experimentation vs. Innovation
49:00 Closing Thoughts and Contact Info
50:30 Outro
Links From The Show
Transcript
In the spotlight: Our most popular episodes
Listen up: Our latest discussions
Hear the hottest takes on data science and AI.
Get the latest episodes in your inbox
Never miss an episode of High Signal by signing up for the Delphina newsletter.