Michael Jordan on The Next Evolution of AI: Markets, Uncertainty, and Engineering Intelligence at Scale

UC Berkeley
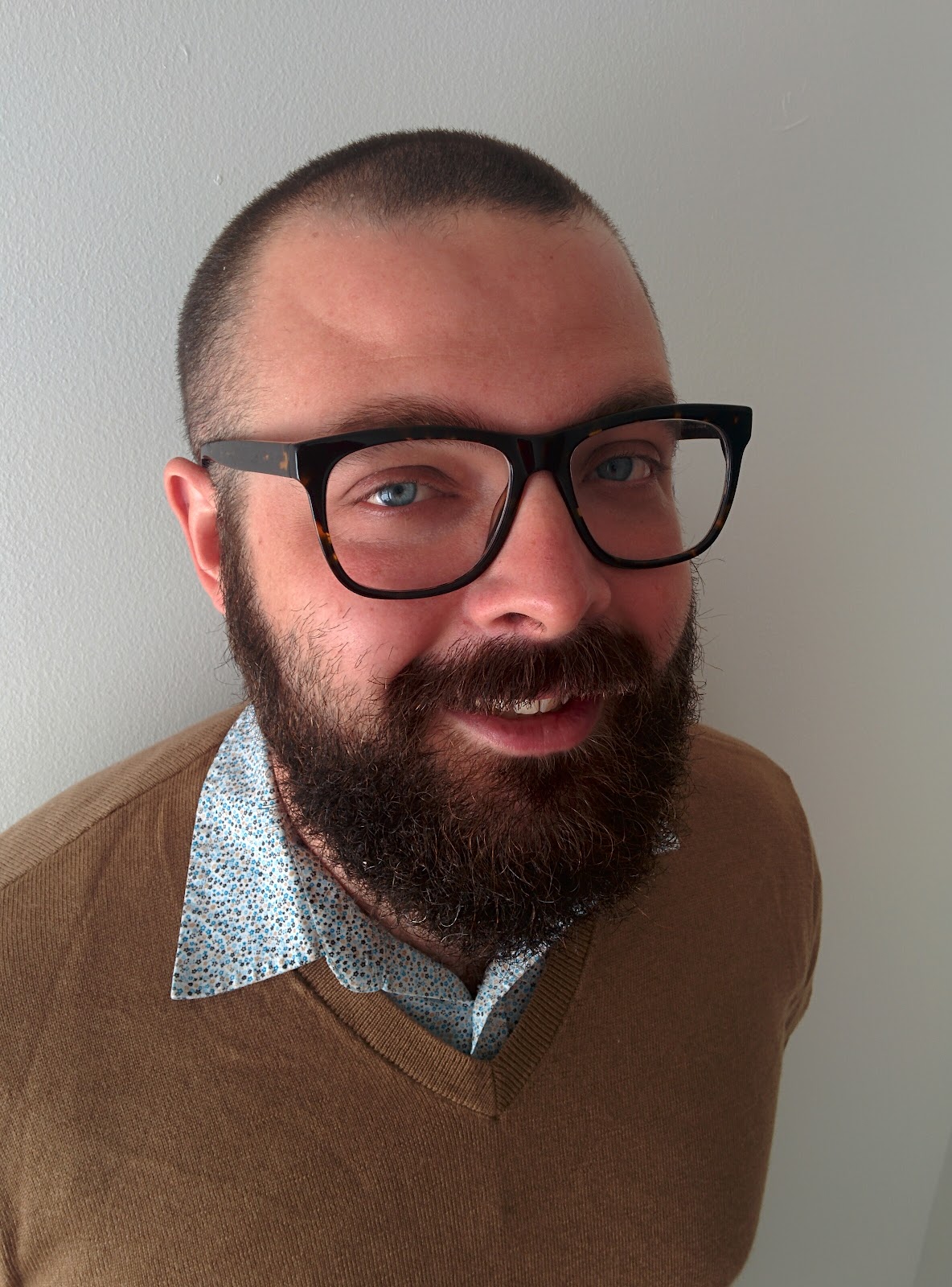
Delphina
Hugo Bowne-Anderson is an independent data and AI consultant with extensive experience in the tech industry. He is the host of the industry podcast Vanishing Gradients, where he explores cutting-edge developments in data science and artificial intelligence.
As a data scientist, educator, evangelist, content marketer, and strategist, Hugo has worked with leading companies in the field. His past roles include Head of Developer Relations at Outerbounds, a company committed to building infrastructure for machine learning applications, and positions at Coiled and DataCamp, where he focused on scaling data science and online education respectively.
Hugo's teaching experience spans from institutions like Yale University and Cold Spring Harbor Laboratory to conferences such as SciPy, PyCon, and ODSC. He has also worked with organizations like Data Carpentry to promote data literacy.
His impact on data science education is significant, having developed over 30 courses on the DataCamp platform that have reached more than 3 million learners worldwide. Hugo also created and hosted the popular weekly data industry podcast DataFramed for two years.
Committed to democratizing data skills and access to data science tools, Hugo advocates for open source software both for individuals and enterprises.
Key Quotes
Key Takeaways
1. AI Terminology and Augmenting Human Intelligence
- The term "artificial intelligence" originally contrasted with "cybernetics" and often misrepresents the goal of AI today, which is more about augmenting human intelligence than replacing it.
- The focus should shift towards "intelligence augmentation."
2. Machine Learning’s Real-World Success
- Machine learning’s breakthroughs in fields like supply chains, fraud detection, and recommendation systems have been driven by large-scale statistical systems rather than traditional logic-based AI.
3. Planetary Scale AI Systems
- AI systems now operate at a planetary scale, making real-time decisions with massive data (e.g., global logistics, healthcare).
- While powerful, these systems face challenges, particularly in managing uncertainty and adapting to dynamic environments.
4. The Triangle of Machine Learning, Computer Science, and Economics
- Future AI development requires the integration of machine learning, computer science, and economics.
- Intelligent systems must also incorporate incentives and economic principles to work effectively in complex environments.
5. Managing Uncertainty
- AI systems often fail to manage uncertainty, leading to overconfidence.
- Handling uncertainty is crucial, especially in high-stakes environments like healthcare.
- Mike’s concept of "prediction-powered inference" bridges predictive models with reliable statistical tools to improve decision-making.
6. Failure Modes in Long-Term Systems
- AI systems can fail over long timescales when they don’t account for changes in the environment, data drift, or shifts in measurement devices, leading to poor decisions in critical areas like healthcare and logistics.
7. Prediction-Powered Inference
- This method combines generative AI models with statistical inference to improve hypothesis testing and scientific research, ensuring more reliable outcomes by managing uncertainty and bias in AI predictions.
8. Three-Way Markets for Sustainable Business Models
- Platforms like United Masters, which create three-way markets (e.g., between musicians, brands, and listeners), show how AI can support sustainable business models by managing interactions and aligning incentives
9. Avoiding AI Hype and Science Fiction Narratives
- The obsession with AGI (artificial general intelligence) distorts the conversation around AI.
- Instead of chasing sci-fi visions of AI, focus should be on building practical tools that augment human capabilities and solve real-world problems.
10. Intelligence Engineering as a New Discipline
- Mike calls for a new discipline—"intelligence engineering"—that focuses on building adaptive, scalable systems integrating economic principles, machine learning, and human decision-making, much like the rise of civil or chemical engineering in the pas
11. Designing Systems That Integrate Economics
- AI systems must incorporate market dynamics, incentives, and scarcity to function effectively at scale.
- Building intelligent systems requires understanding economic systems, not just technical ones.
12. Ethical and Societal Impact
- While not explicitly discussed, the underlying theme emphasizes the need for ethical engineering in AI, ensuring that systems are built to enhance human welfare, manage uncertainty, and avoid harmful impacts in critical sectors like healthcare and global logistics.
AI’s future lies not in creating "superintelligent" machines, but in building resilient, adaptive, and ethically responsible systems that operate at a planetary scale, augmenting human intelligence and addressing complex societal and economic challenges.
You can read the transcript here.
Timestamps:
00:00 Introduction to High Signal
00:17 The Evolution of AI with Michael Jordan
00:37 The Future of AI: Combining Fields
00:55 Challenges in AI: Uncertainty and Real-World Impact
01:22 Personal Stories and Broader Impacts
01:34 Clip: The Evolution of AI
02:09 The Intersection of Fields: Machine Learning, Economics, and Computer Science
04:45 Introducing the Delphina Team
08:05 Interview with Michael Jordan Begins
08:45 Historical Perspectives on AI
10:48 Intelligence Augmentation vs. Artificial Intelligence
12:18 Supply Chains and Machine Learning
13:39 The Role of Data in AI
21:28 The Importance of Provenance in AI Predictions
24:42 The Wisdom of Crowds and AI
33:49 Generative AI and Its Applications
38:02 Monte Carlo Experiment and Error Bars
38:36 AlphaFold's Limitations
39:32 Improving Prediction Accuracy
41:07 Self-Driving Cars and Error Bars
43:54 United Masters: A Three-Sided Market
50:18 Statistical Contract Theory
53:38 Uncertainty in Decision Making
01:09:25 The Future of Intelligent Systems
Links From The Show
Transcript
In the spotlight: Our most popular episodes
Listen up: Our latest discussions
Hear the hottest takes on data science and AI.
Get the latest episodes in your inbox
Never miss an episode of High Signal by signing up for the Delphina newsletter.