Chris Wiggins on What Lies Beyond Machine Learning and AI: Decision Systems and the Future of Data Teams
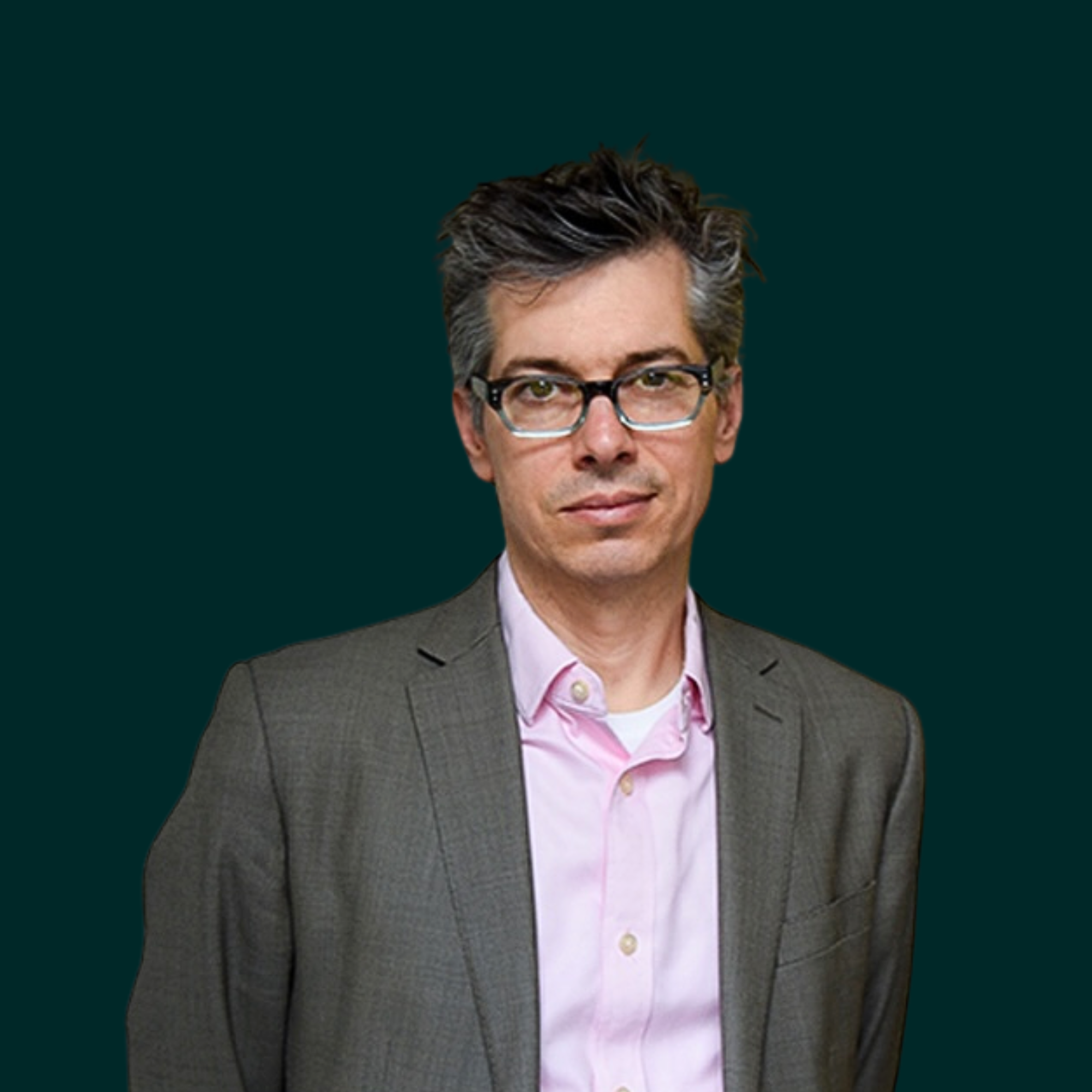
New York Times
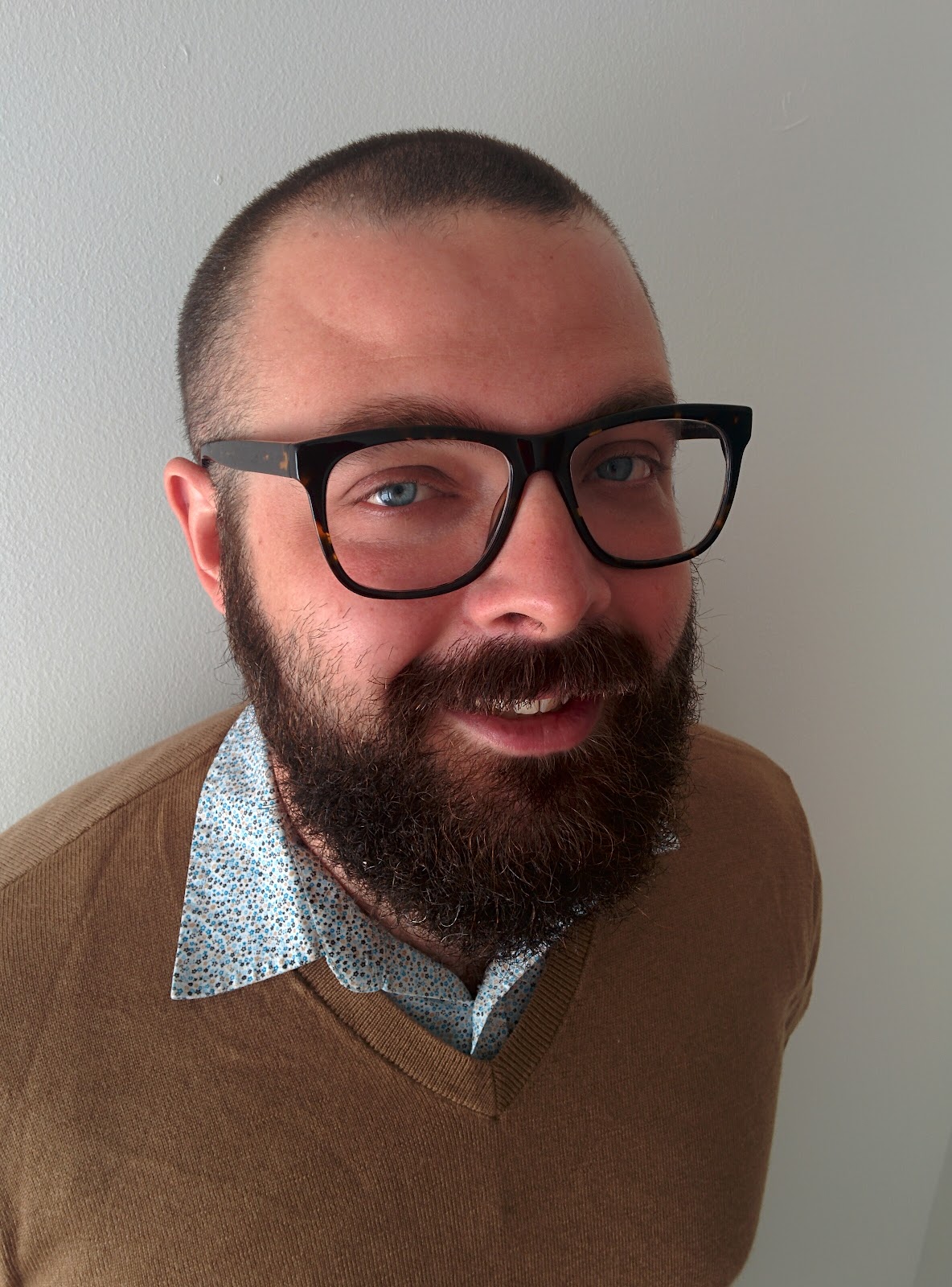
Delphina
Hugo Bowne-Anderson is an independent data and AI consultant with extensive experience in the tech industry. He is the host of the industry podcast Vanishing Gradients, a podcast exploring developments in data science and AI. Previously, Hugo served as Head of Developer Relations at Outerbounds and held roles at Coiled and DataCamp, where his work in data science education reached over 3 million learners. He has taught at Yale University, Cold Spring Harbor Laboratory, and conferences like SciPy and PyCon, and is a passionate advocate for democratizing data skills and open-source tools.
Key Quotes
Key Takeaways
Key Takeaways
- From Prediction to Prescription: Driving Action with Data Science
- Prediction is not the end goal—true value comes from prescribing the right interventions to influence outcomes.
- Chris states: “The question is not just how you can predict what's going to happen in the absence of treatment. The question is how can you prescribe the optimal treatment in order to drive some event that you want.”
- Building Data Science Teams That Align with Organizational Goals
- Success in data science requires empathy, communication, and alignment with partner teams across the organization.
- Chris highlights: “Other teams are the keys to getting things done at scale.” He stresses that understanding others’ goals drives adoption and impact.
- The AI Hierarchy of Needs: Focus on Fundamentals Before AI
- Start with strong data engineering, logging, and observability before building advanced models.
- Chris underscores: “If the input falls down and there’s no observability, what’s the point of fancy AI?” Citing Monica Rogati’s framework, he emphasizes the importance of reliable foundations.
- Empathy as a Critical Skill for Data Scientists
- Data scientists must communicate the value of their work clearly to ensure organizational alignment.
- Chris advises: “Make sure that everything you're working on, you could explain to anybody in the organization why it's an important thing to work on.” This clarity drives trust and buy-in.
- From Proofs-of-Concept to Production Systems
- One-off prototypes may be provocative, but the real challenge lies in systems integration and scaling solutions across the organization.
- Chris explains: “Doing one-off fancy artificial intelligence can be very useful as a provocation... But it’s not the same thing as a prototype, build one complete solution, then work on systems integration and process integration.”
You can read the full transcript here.
00:00 Introduction to Chris Wiggins' Journey
00:07 Building Data Functions at The New York Times
00:48 Early Challenges and Evolution
01:07 The Importance of Prescriptive Analytics
02:07 Optimization and Personalization
03:25 AI Hierarchy of Needs
04:01 Effective Data Science Teams
08:02 Chris Wiggins' Career Journey
14:01 Building a Data Function at The New York Times
16:44 Predictive to Prescriptive Analytics
22:03 History of Data Science
23:46 Statistics and Power
27:00 Building a Data Function: Practical Insights
39:42 Choosing the Right Tool for the Job in Machine Learning
40:35 Causal Inference and Reinforcement Learning
42:02 The Importance of Randomized Control Trials
43:59 Principal Component Analysis and Data Function Priorities
46:47 Empathy in Data Leadership
52:42 Generative AI in Education
58:05 Interdisciplinary Collaboration in Academia and Industry
01:10:34 Future of Data Science at The New York Times
01:15:11 Closing Thoughts and Advice for Data Science Leaders
Links From The Show
Transcript
In the spotlight: Our most popular episodes
Listen up: Our latest discussions
Hear the hottest takes on data science and AI.
Get the latest episodes in your inbox
Never miss an episode of High Signal by signing up for the Delphina newsletter.