Andrew Gelman on Fooling Yourself Less: The Art of Statistical Thinking in AI
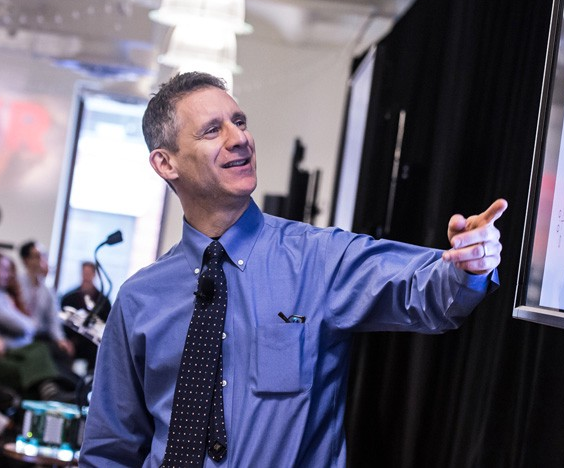
Columbia University
Andrew Gelman is a professor of statistics and political science at Columbia University, recognized with multiple awards from the American Statistical Association, the International Society of Bayesian Analysis, and the Council of Presidents of Statistical Societies. He is the author of numerous notable books, including "Bayesian Data Analysis" and "Regression and Other Stories."
His research spans topics such as voting behavior, campaign polling variability, incumbency effects, death sentence reversals, police stops, and a variety of statistical challenges in public health and social sciences.
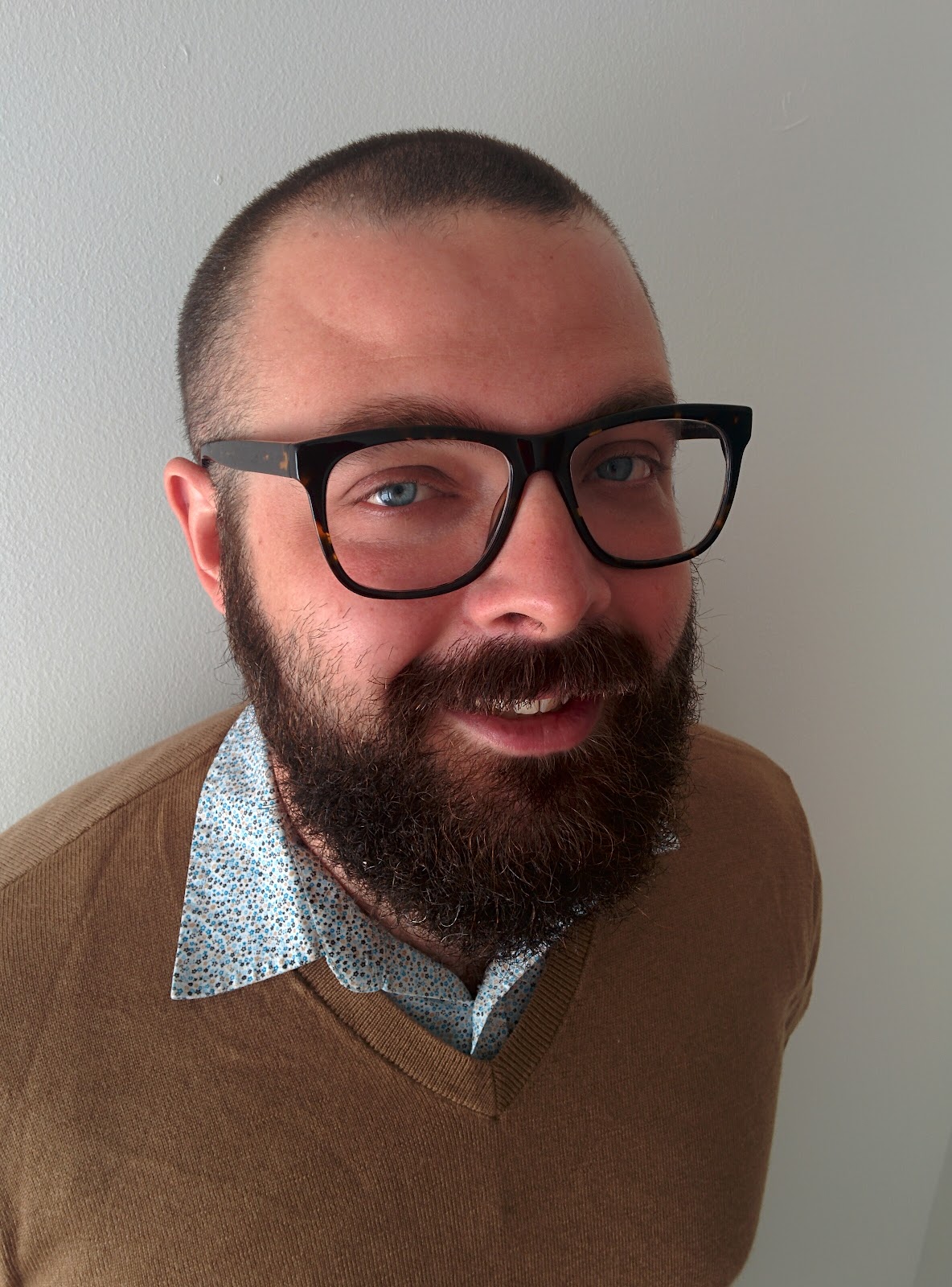
Delphina
Hugo Bowne-Anderson is an independent data and AI consultant with extensive experience in the tech industry. He is the host of the industry podcast Vanishing Gradients, where he explores cutting-edge developments in data science and artificial intelligence.
As a data scientist, educator, evangelist, content marketer, and strategist, Hugo has worked with leading companies in the field. His past roles include Head of Developer Relations at Outerbounds, a company committed to building infrastructure for machine learning applications, and positions at Coiled and DataCamp, where he focused on scaling data science and online education respectively.
Hugo's teaching experience spans from institutions like Yale University and Cold Spring Harbor Laboratory to conferences such as SciPy, PyCon, and ODSC. He has also worked with organizations like Data Carpentry to promote data literacy.
His impact on data science education is significant, having developed over 30 courses on the DataCamp platform that have reached more than 3 million learners worldwide. Hugo also created and hosted the popular weekly data industry podcast DataFramed for two years.
Committed to democratizing data skills and access to data science tools, Hugo advocates for open source software both for individuals and enterprises.
Key Quotes
Key Takeaways
1. Statistics vs. Data Quality
Data quality and representativeness take precedence over statistical methods in data science. While statistical techniques are important for quantifying uncertainty and adjusting for non-representativeness, they are secondary to ensuring high-quality data.
2. The Importance of Computer Skills in Data Science
Computational skills, such as being able to handle data and use tools, are often more important than math skills in data science. While math provides useful insights, data scientists need a balance of both to succeed.
3. The Power of Simulation for Learning Statistical Concepts
Simulations are a practical way to teach statistical concepts, like the central limit theorem, in an accessible manner. Simulation allows people to "see" statistical principles emerge in ways that pure mathematics often cannot.
4. Polling and Probabilities: Simulating Elections
The concept of calculating the probability of a vote being decisive in an election was explained, demonstrating how empirical, statistical modeling, computer simulation, and mathematical understanding can combine to address real-world problems like election predictions.
5. First Principles Thinking in Experimental Design
Through an example about education experiments, the importance of first-principles thinking in designing experiments was emphasized. Estimating effect sizes and using simulations can help anticipate realistic outcomes before gathering data.
6. The Power of Mental Simulation in Causal Inference
The value of mental simulations and causal inference in data science was discussed. When estimating the impact of interventions or treatments, data scientists must go beyond just estimating parameters and instead focus on creating models for potential outcomes.
7. Polling Challenges and Misconceptions
Polling has not become less accurate over time. Non-sampling errors have always existed, but people's expectations for precision have increased. In close elections, the inherent uncertainty makes it difficult to predict outcomes with extreme precision.
8. Communicating Uncertainty and Quantitative Thinking
Communicating uncertainty, particularly in probabilistic terms, is challenging. Using examples like disease testing, it was shown how rare events and probabilistic thinking can lead to unintuitive conclusions, stressing the importance of clear communication in data science.
9. Generalization as a Core Statistical Concept
Generalization is crucial in data science—whether generalizing from sample to population, control group to treatment group, or from data to underlying constructs. This concept is key but often under-emphasized in statistics.
10. Simulation as a Tool for Better Experimental Design
Simulating data before collecting it improves experiment design by forcing scientists to confront assumptions about populations and sampling mechanisms, leading to better insights.
11. Avoiding the Pitfalls of Methodological Attribution
There’s a danger in attributing success too much to a specific statistical method without recognizing the importance of the underlying model. Statisticians and data scientists should focus on understanding when methods fail to grasp their true applicability.
12. The Rationality of Voting in Elections
Voting can be rational, even in large elections, by considering the small probability of decisiveness and the large potential societal benefit. This demonstrates how seemingly irrational behavior can have a rational basis when viewed from a broader perspective.
13. Fooling Ourselves in Data Science
Statisticians and data scientists often fool themselves by overstating the significance of their results or methods. Approaches like replication studies and maintaining a healthy skepticism about one's own results are key to reducing self-deception.
14. Applying Causal Inference in Data Science
Causal inference is a predictive exercise, comparing potential outcomes under different treatments. Understanding this comparison is crucial for making meaningful inferences in data science.
Timestamps:
00:00 Introduction to High Signal with Andrew Gelman
00:30 The Practical Side of Data Science
01:07 Simulating Data Before Gathering
01:47 Thinking Like a Coder in Statistics
02:20 The Importance of Comparison in Statistics
02:52 Meet the Team at Delphina
05:21 Starting the Interview with Andrew Gelman
05:43 Data Quality and Representativeness in Data Science
07:05 The Role of Computer Skills in Data Science
08:55 The Power of Simulation in Statistics
16:41 Designing Effective Experiments
24:00 Causal Inference and Predictive Statements
26:38 The Rationality of Voting
30:33 Rational Voting and Local Elections
31:58 Theoretical Models and Real Voting Behavior
35:52 Polling Accuracy and Challenges
40:35 Understanding Uncertainty in Statistics
46:16 Future of Statistical Techniques
53:31 Avoiding Self-Deception in Data Science
55:01 Practical Tips for Data Scientists
01:00:09 Concluding Thoughts and Farewell
Links From The Show
Transcript
In the spotlight: Our most popular episodes
Listen up: Our latest discussions
Hear the hottest takes on data science and AI.
Get the latest episodes in your inbox
Never miss an episode of High Signal by signing up for the Delphina newsletter.